Understanding the Support Experience
M. Fraser, Sr. UX Researcher
J. Swartz, Assoc UX Researcher
R. Zelaya, CX Researcher
Agents as a function of CARE were seen as a pain point in the following ways
- Financially
- Time taken to resolve cases was higher than average
- tNPS scores were unsatisfactory
A solution suggested was to redesign the agent dashboard. A list of features, possible designs and improvements and ways of understanding the process were seen as the potential outcomes. Since we had very little knowledge of what the day to day needs and work of our care agents, so designed a research project getting at this knowledge
Initial exploration
Reviewing the care rep performance data uncovered an unexpectedly long amount of time both during and after the care calls. We had expected the average call to be 5-10 minutes but the average call was 12 minutes or more. In addition, resolution rates were lower than expected possibly meaning repeat calls by the customer to resolve their issue leading to higher than expected call volume.
Methods:
We used a primary and secondary methodology to identify impacts on time.
The primary methodology was contextual in nature. We shadowed and interviewed agents during the process of answering calls. We attempted to understand their normal work flow and understand the pain points associated with the process. We investigated tools, dashboards, and systems used to handle calls. The end goal here was to quantify product issues and customer facing issues.
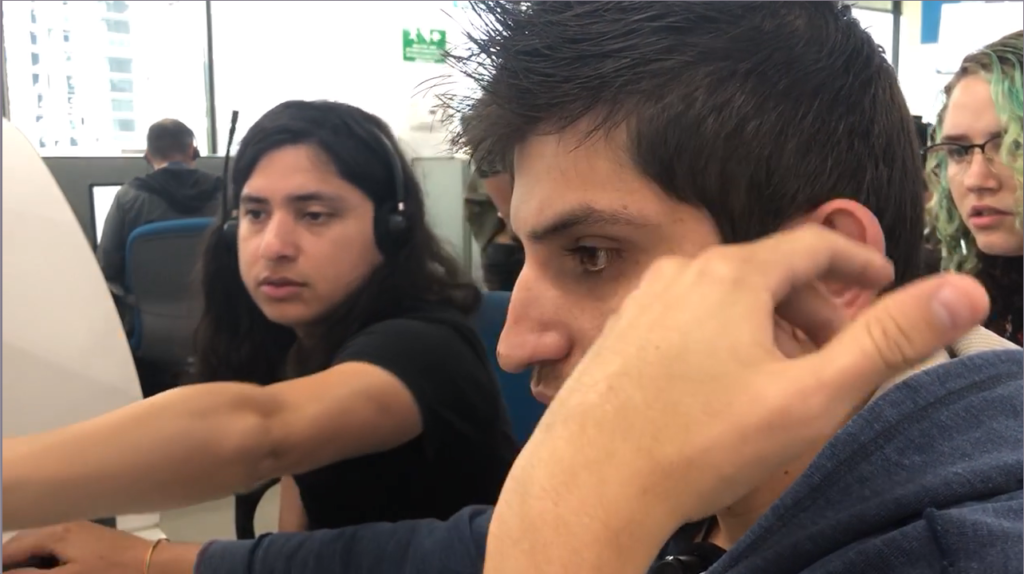
The second was a cafe study. We chatted with agents over coffee one-on-one and spoke about their day-to-day experiences, specifically asking about the pros and cons of working at the call center. This was done to understand the motives behind their process and work life. We interviewed the agents outside of the work environment and asked about their day to day lives, commuting, living situation etc. We took them out of the work environment to give them a space to vent about their jobs and lives without a manager’s presence influencing their answers or speech. We wanted to understand their lives in order to streamline their workspace thus ensuring a greater ability to manipulate dashboards and other tools.
Results
Affinity mapping revealed a few themes.
First, we noticed there were organizational processes which increased customer effort. For example: our partners in costa rica would hold a required manager’s meeting each morning for 15-20 minutes. However managers were part of a required business process for refunds approval. If a customer was unlucky enough to call during this time they would have to wait longer for help with a simple business process.
We also learned about frustrations with tools the agents were equipped with. For instance for security reasons agents weren’t allowed able to download or save files. So if a customer wanted a report of their usage, a supervisor or manager had to download the file to email it to the customer. This was problematic as agents couldn’t take notes of their calls to save for follow ups. In short, agents were denied simple procedural behaviors that would allow them to do their job quickly, creating a longer time on task.
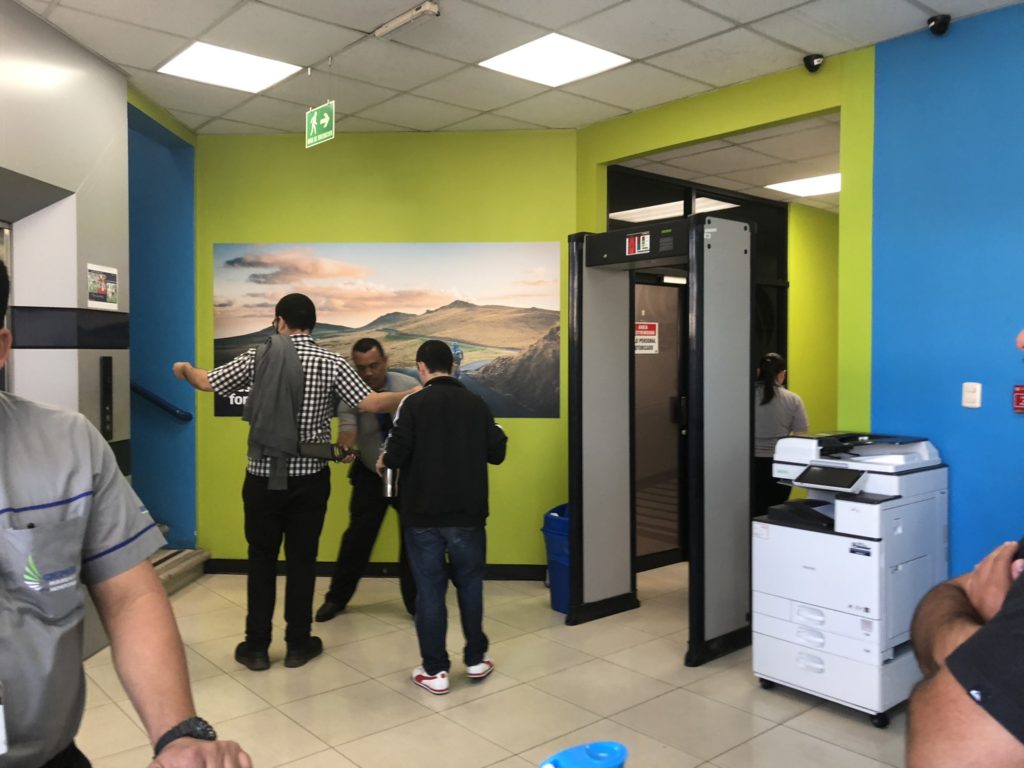
Additionally, we noticed the work culture at the partner site led to distrust. Regular security checks, the aforementioned security restrictions and a lack of mobile devices (needed to troubleshoot mobile applications). We recommended a renegotiation of contract with our near shore partners, or a return to having internal customer care teams.
Outcomes
Customer care operations are moving back in house. This affords greater control over tools and processes, so agents and customers are set up for a lower effort experience.
Agents are now given the same permissions as supervisors, and are trained to use their own judgment for refunds and other issues that would have required supervisor intervention.
FInally a culture of trust is fostered. Agents are now a part of our company and not external resources hired for a 3rd party contract. This creates a sense of belonging and trust in our representatives who are on the front lines of our customer experience.