M. Fraser, Sr. UX Researcher
J. Swartz, Assoc UX Researcher
R. Zelaya, CX Researcher
Initial Situation
The Customer Care team had no insight into:
- Why customers were calling support
- If issues required agent touch or could be self serviced
- Why tNPS scores were unsatisfactory
When we began this project, the customer care team had little insight into why people were calling in to the CARE center.
We needed to target the top call drivers
Initial exploration
Examining the data, we found that 75% of the issues fell into just 3 categories (Account Set Up, Technical Issue, or Other) indicating that the categories were not specific enough or the agents were fatigued with replying in such a short time. The only categorization was being done by the agents in a 30 second allotted time post call. The list of potential issues Agents were to choose from, was extremely long and often not specific to what the agent was working on.
Because of these confounds, it was impossible to accurately measure call volume by specific issues.
We had little insight into which issues absolutely required agent assistance to handle vs. issues that customers could be self serviced. The building support of articles for issues resulted in customers eventual still having to call in for answer, e.g. Customers would read through articles first instead of being directed to call immediately, increasing overall effort and frustration on the customers part.
Finally, there were problems routing customers to correct channels. Thus, customers sent in multiple emails about an issue before finally being directed by an agent to call the support line. This led to higher operating costs and lower tNPS scores
Methods
We set up a multi-pronged approach to identify problems and address potential recommendations to the teams responsible for each problem
First Phase: Customer Listening
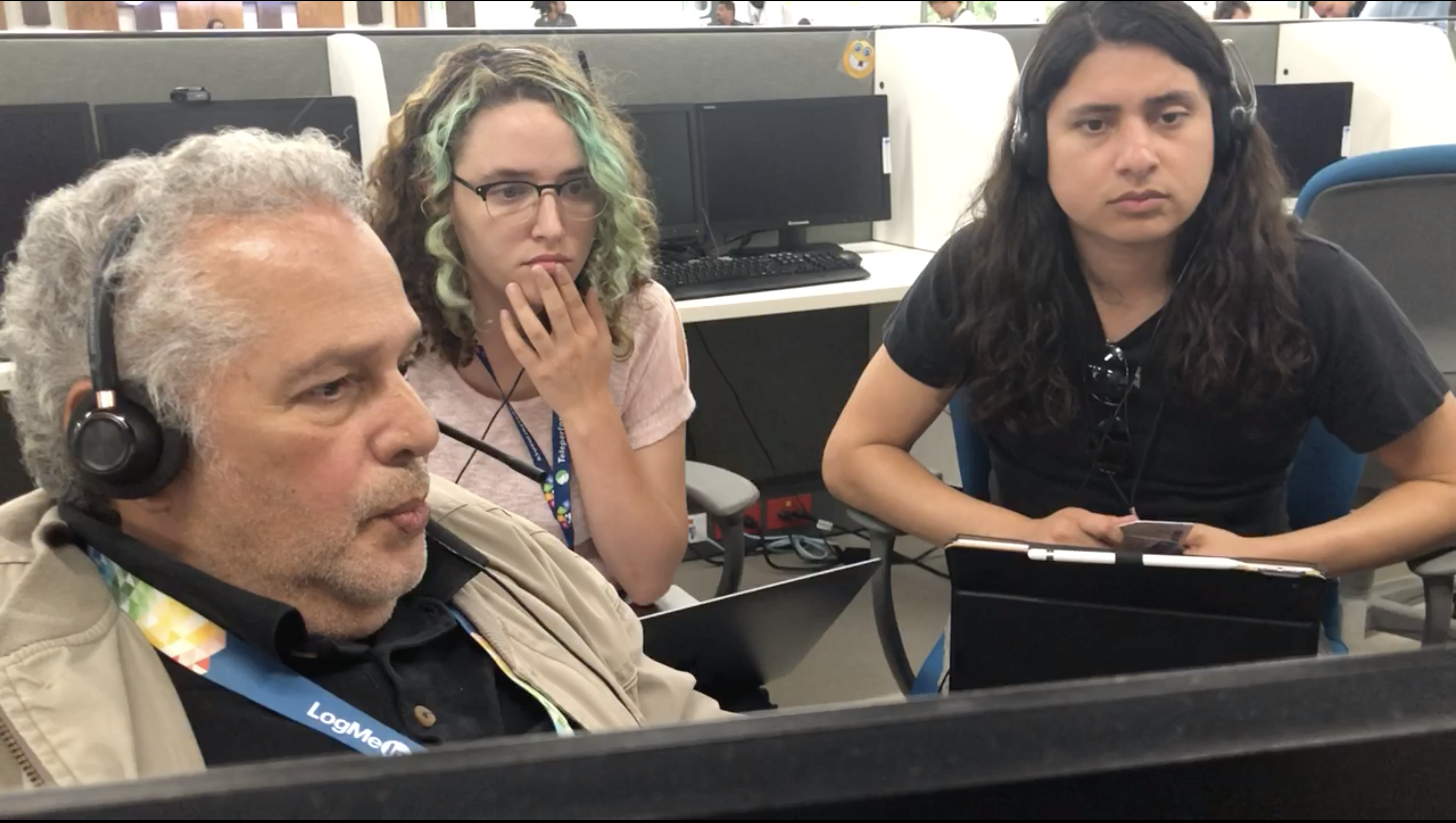
Auditory observation in order to have a form of context
The purpose of this was to create logical categories to sort the incoming calls by to determine navigation on the self-help pages. We used the largest Business Unit to help us set a scaffold of these issues as well as who was most likely to have them.
Listening to 350 customers, we identified groups of customer segments by their roles: Administrators, Billing administrators, event organizers, attendees, and prospective customers.
Listening to hours of customer care calls led to unexpected insight on the agent’s process – there were many instances of agents using:
- work arounds,
- running into problems that should have been easy to fix,
- and generally delaying the time to resolution
From this project we iterated and planned a project to understand the agent experience in true contextual inquiry.
Second Phase: Issue Gathering, Affinity Mapping, Card Sorting
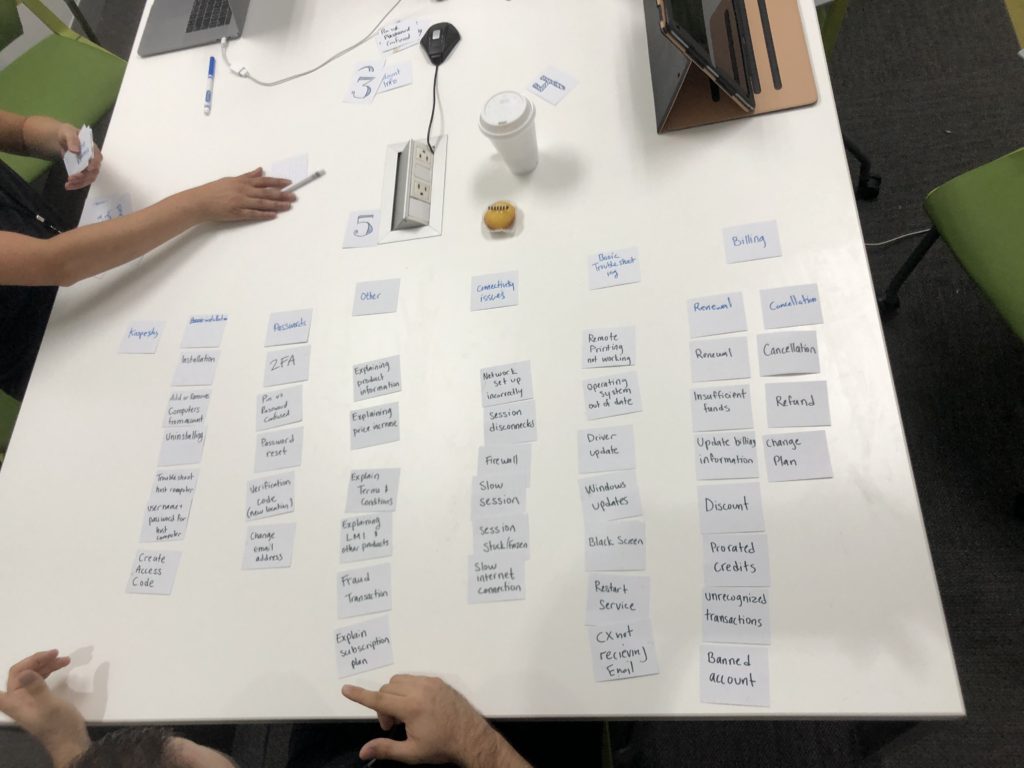
The purpose of this phase to discover an exhaustive list of issues that the agents handle.
An issue gathering workshop with groups of agents from other different business units was held We asked agents to affinity map the issues as a group into different categories that they determined as essential.
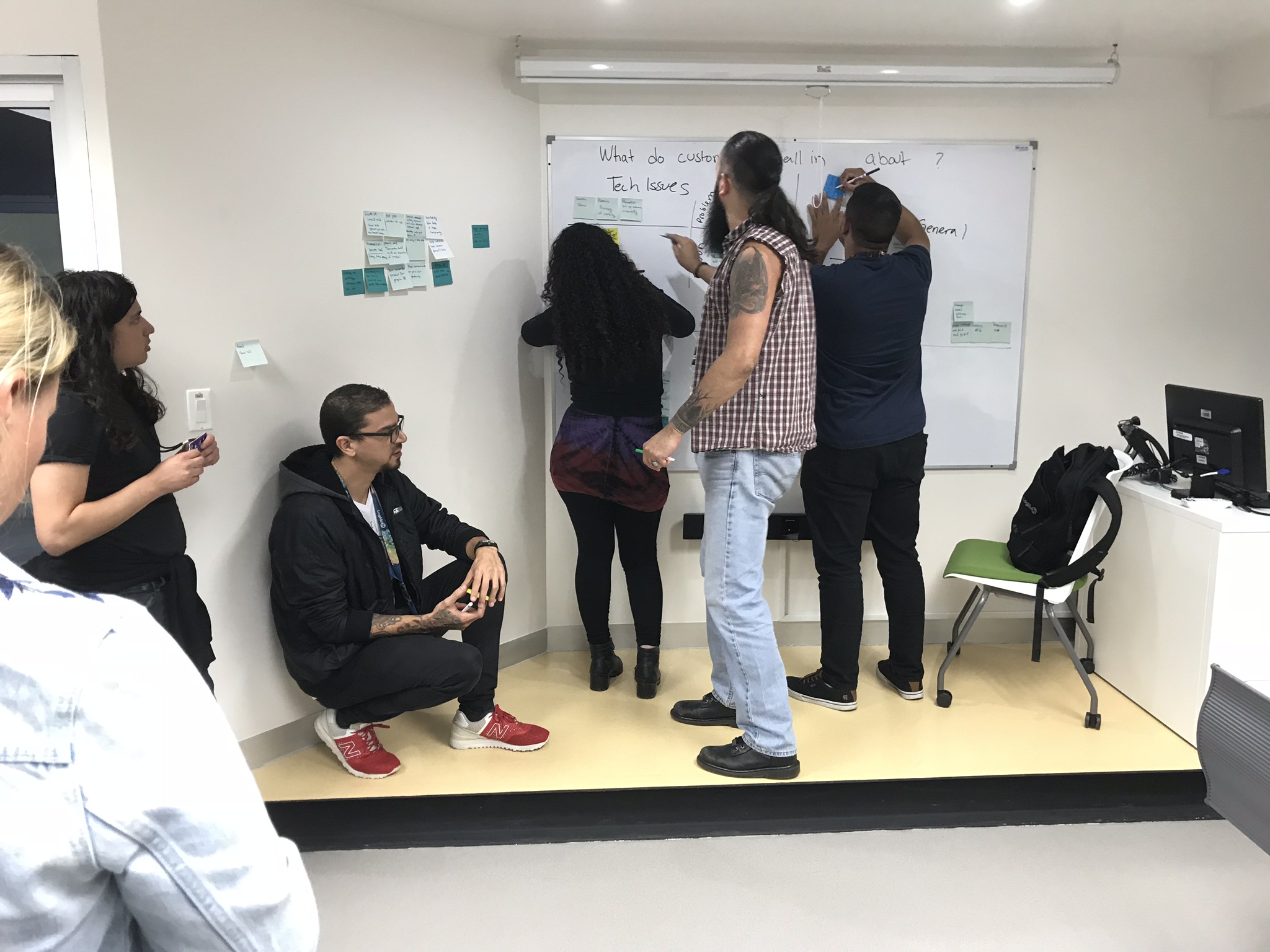
To discover the hierarchy of information, a card sort with individual agents from two of the Business Units was run. (N= 12, n=6)). We also asked agents to sort issues by” “self-serviceable” or “agent touch”
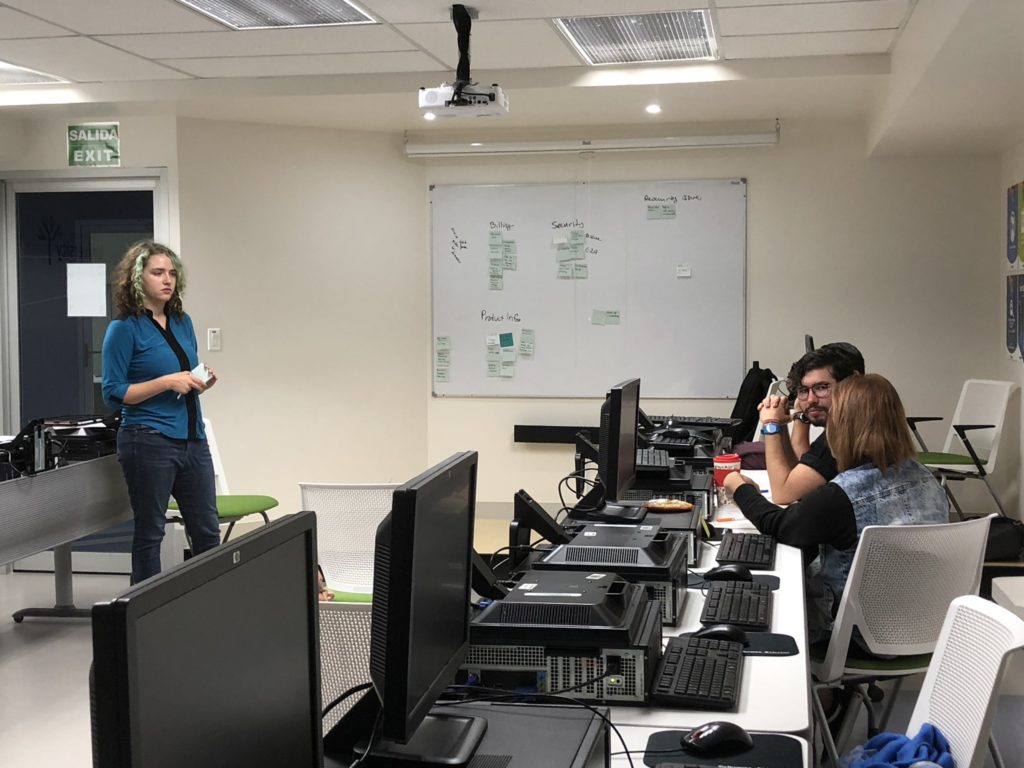
We ran the same exercise with the Partner Management teams from all three Business Units, and compared the information and issues listed from all groups. In addition, the partner management team also ran an issue-to-effort sort. Issues were sorted by perceived level of effort on the X axis and whether the issue was self-serviceable or required agent touch on the Y axis.
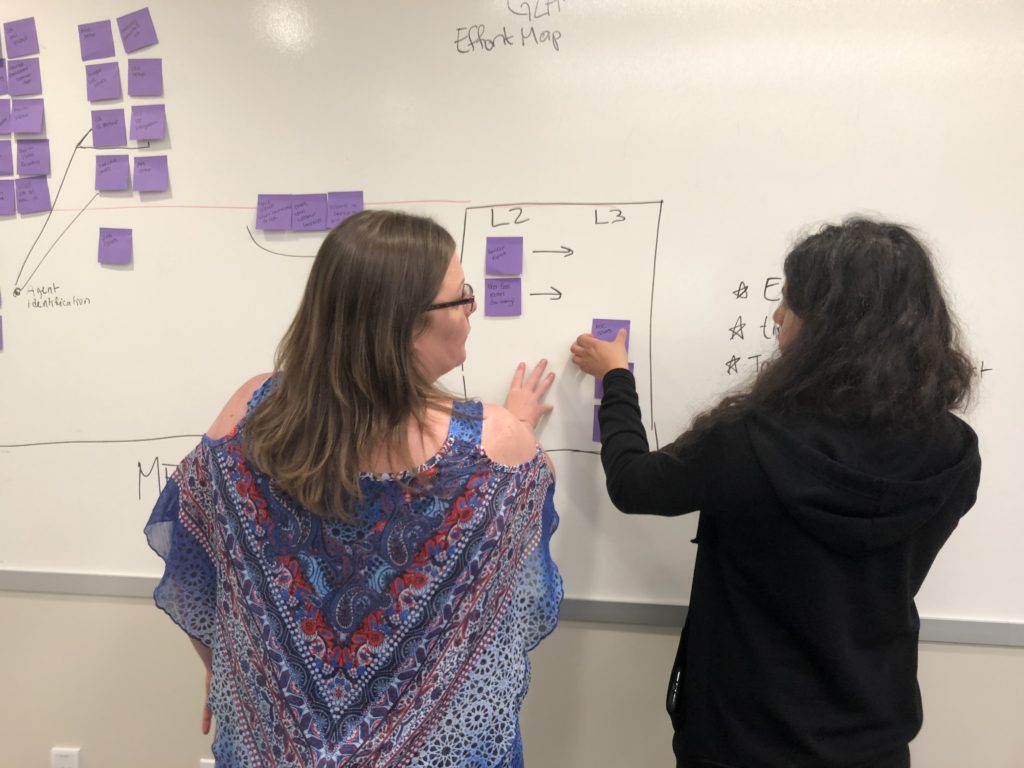
We uncovered low-effort agent touch issues that we could expose to customers for self-service. We also discovered high-effort customer-facing issues that we could provide more targeted support and education around on the support website.
Third Phase: The Issue Mapping Spreadsheets
This phase allowed us to organize content creation in an issue-first strategy for the support site by uncovering what issues didn’t have support content while.
Data from past projects, Gartner’s industry best practices, and our own content from our self-help page, A spreadsheet with all the issues gathered was categorized into self-service or agent touch issues, level of effort, the resolution job that the the issue fell into, and links to any self-help article we had on the topic.
*note – each of the 21 products had it’s own spreadsheet.
Currently these spreadsheets are being used as a content map for BOLD360ai (the chatbots for each product) and Clarabridge modeling (our NLP that looks at call transcripts to identify customer issues).